The move towards AI in labour forecasting within the retail sector underscores a strategic pivot from reliance on traditional, rule-based models to embracing the adaptability and precision offered by machine learning and AI technologies. This approach promises not only to refine labour forecasting but also to catalyse a broader organisational transformation in how data-driven decisions are made and trusted. But is it really better, and what does an implementation mean?
by Fredrik Kangevall, Fredrik Moeschlin & Karl Svensson
The retailers’ challenge and how we used to “solve” it
In the heart of every retail warehouse lies a critical challenge: accurately predicting workload and labour needs. This demand is intricately tied to sales, yet sales patterns are far from static, often following seemingly irregular trends. Traditionally, we’ve leaned on manual observations or, at best, statistical models to forecast these requirements;, screening historical sales data and attempting to account for outliers by imposing predefined rules.
Recalling our experience of implementing a forecasting engine for a leading grocery retailer in the Nordics a decade ago, this traditional approach was our starting point. For instance, we observed that one of the primary drivers of sales was the timing of salary payments, particularly around the 25th of each month. However, we quickly realised that our forecasts needed to consider a myriad of other factors too, such as seasonal events like Easter (which varies in date each year), ongoing campaigns, and even geographical variations. The challenge arose when unforeseen events occurred outside of our set assumptions, resulting in either overstaffing or understaffing — with higher stress, increased costs, lower quality and grumpy customers as consequences.
Herein lies the fundamental difference in utilising AI for labour forecasting:
- AI does not rely on a fixed set of assumptions to explain behaviours.
- AI continuously adapts its rules and parameters over time based on new data and insights.
But the burning question remains: does AI forecasting yield superior results, and what are the challenges that need to be handled during the implementation? To answer this, let us review two real-world cases.
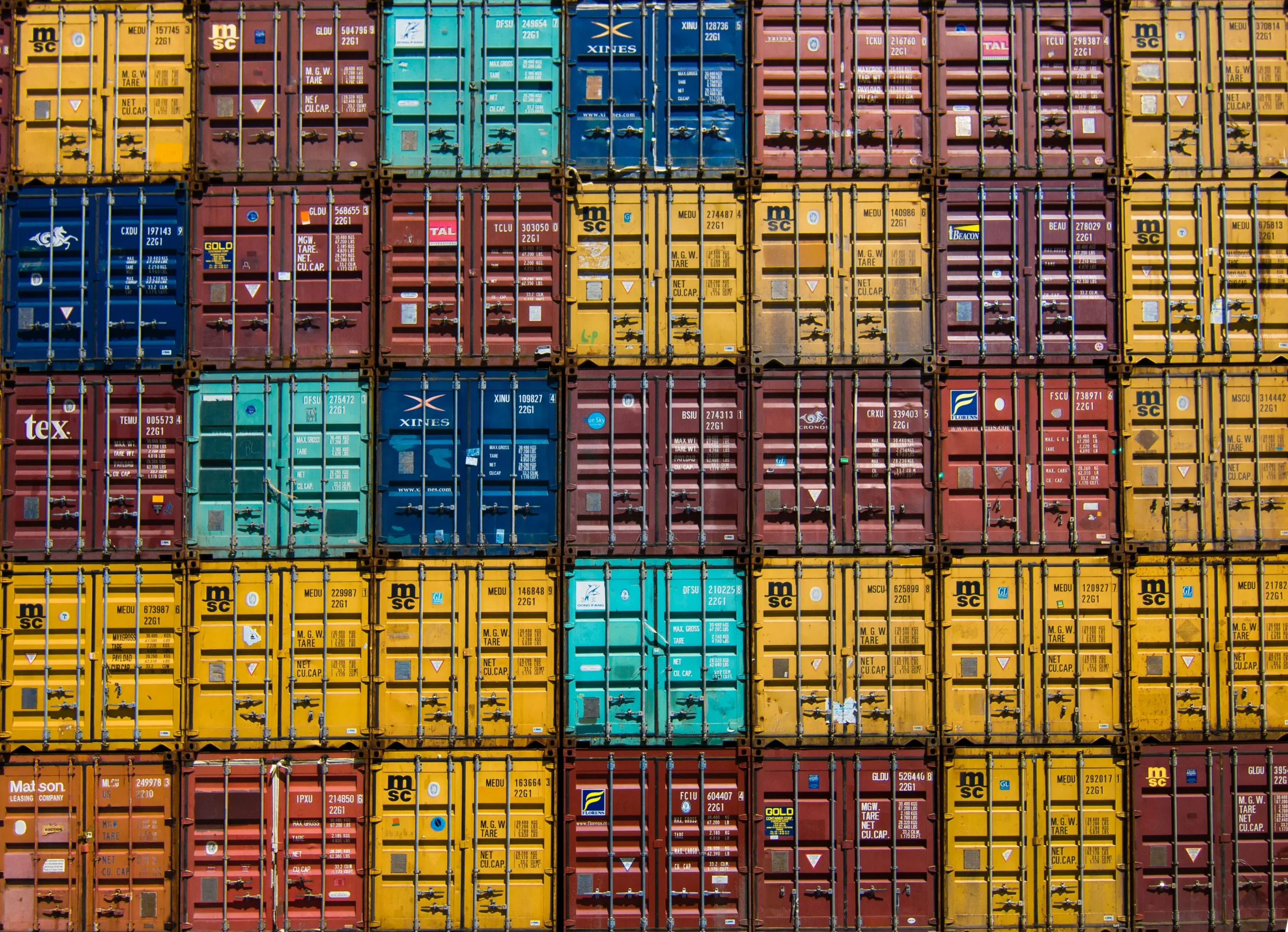
An alternative approach
In 2019, a leading Swedish company in the online food retail market faced a pressing challenge: “How do we accurately predict sales patterns for our goods?”. The proficiency of the existing manual approach was sub-par, resulting not only in over and under staffing the warehouse, but also in wasting goods and transport allocations. Perishable goods, like fruits, vegetables etc., had to be discarded, redundant storage space had to be allocated to buffer non-perishable goods, and the lorries were either overflowing or almost empty. The root cause was unexpected variations from normal customer behaviour.
At the close of 2023, the situation was similar at a leading Swiss company providing solutions and products for professional craftsmen and DIYers. They embarked on a transformative journey to overhaul their warehouse labour forecasting system. With a diverse sales portfolio spanning both a physical store network and an online platform, the company faced significant hurdles in determining the optimal staffing levels required at various junctures. The challenge was not merely the fluctuations in activities such as receiving, picking, packing, and shipping. On certain days, despite having the seemingly appropriate number of staff on duty, who were all performing efficiently, the workload appeared overwhelming. In pursuit of enhancing forecasting precision and operational efficiency, the retailer turned its sights towards the capabilities of AI technology.
Setting up an AI project
In collaboration with us, both companies decided to explore the potential benefits of AI. Central to our strategy was the rapid deployment of a Minimum Viable Product (MVP) to either validate or refute our approach. Our setup aimed for simplicity and autonomy from extensive IT infrastructures to bypass the pitfalls of time-intensive tasks that offer little immediate value. Our objectives were clear:
- Could AI surpass the existing forecasting and planning and
- would the staff trust the AI tools?
It’s crucial to recognise that achieving superiority with the AI model in the initial phase didn’t necessitate its perfection across all conceivable scenarios. Instead, our goal was to identify a solution that could address 80% of the forecasting challenges effectively. By doing so, we could liberate significant resources to tackle the remaining 20% of the issues. The project was leanly staffed, featuring a dedicated key stakeholder from the retail side and a compact team maintaining direct communication with the client, ensuring a focused and efficient project progression.
Utilising a blend of historical staffing data, sales records and other pertinent variables, we crafted systems powered by advanced AI models. The models use machine learning techniques to analyse and predict staffing needs.
The most time-consuming aspect, as is often the case, was obtaining and cleaning data. While we strongly believe this will change along the road, the reality remains that many companies today do not have their data organised and ready for immediate use. This necessitates continuous strategic decisions to avoid getting stuck.
The forecasting process itself, however, was markedly quicker. Unlike traditional methods that require the laborious task of building a basic model and then incrementally refining it through the application of new rules, our AI-driven approach saves significant time. Yet, it’s important to note that this efficiency comes at a cost. The AI methodology by many considered a “black box”, making it essential to invest in people management to foster trust in the AI-generated forecasts. While this challenge is not entirely dissimilar to that faced with traditional statistical methods, it represents a notable shift for companies accustomed to manual forecasting processes. The question of accountability when forecasts are inaccurate underscores the need for a cultural adjustment within organisations to build confidence in the AI’s predictions. If suddenly there are 15 unwanted pallets of smelly herring in the warehouse, who is to blame?
Harvesting the benefits
For the Swiss retailer the outcome of the project was very positive. The MVP developed within a couple of weeks showed strong predictive power. During an evaluation period the retailer could clearly see that the forecasts were mostly in range and often spot on. The period had large variations which were handled well by the model, but which were hard to foresee manually. The AI forecast will now allow the retailer to reduce stress level within the work force, allow for optimized resource planning, and facilitate the daily operations.
The Swedish online food retailer saw big improvements compared to the manual approach. Initially, the AI powered predictor worked alongside the manual forecasting personnel, who compared their forecasts with the ones produced by the AI. Consistently observing the AI mimic their predictions during periods of normal customer behaviour and outperforming them during hectic periods gradually increased their level of trust until finally, the AI was allowed to autonomously recommend staffing, purchasing and transports. To increase trust even further, safeguards were installed to highlight extreme recommendations for manual inspection. By installing an AI powered predictor, the company saw savings across the board: optimal staffing levels, less waste, reduced storage space and more efficient transports.
Key take aways
Benefits
- Flexibility and adaptability: AI does not rely on a fixed set of assumptions but continuously adapts to new data, making it more flexible and accurate in forecasting labour needs compared to traditional statistical models.
- Quick implementation of MVP: A focused approach on deploying a Minimum Viable Product (MVP) allows for a quick test of AI’s efficacy over traditional methods without significant IT overhead. This reduces the entrance barrier with lower cost and reduced risk.
- Effective resource allocation: By aiming to solve a significant portion of forecasting challenges effectively, AI frees up resources to focus on refining and tackling the remaining issues, optimising operational efficiency.
- Time efficiency in development: The use of machine learning techniques in AI models reduces the time required to develop and refine forecasting models, bypassing the step-by-step refinement needed in traditional methods.
To consider
- Data availability and readiness: This remains a key enabler for effective AI solutions. Good data availability and quality is a requirement for good AI results.
- Building trust and fostering organisational transformation: Transitioning to AI for forecasting requires significant investment in people management to build trust in AI generated forecasts. This process encourages a cultural shift towards acceptance of automated decision-making and paves the way for a broader organisational transformation towards data-driven practices and advanced analytics.